Anomaly detection based on automatic reverse engineering of CAN automotive packets
Project Summary
Automotive security has gained significant traction in the last decade thanks to the development of new connectivity features that have brought the vehicle from an isolated environment to an externally facing domain. Researchers have shown that modern vehicles are vulnerable to multiple types of attacks leveraging remote, direct and indirect physical access, which allow attackers to gain control and affect safety-critical systems. Conversely, Intrusion Detection Systems (IDSs) have been proposed by both industry and academia to identify attacks and anomalous behaviours. In this paper, we propose CANnolo, an IDS based on Long Short-Term Memory (LSTM)-autoencoders to identify anomalies in Controller Area Networks (CANs). During a training phase, CANnolo automatically analyzes the CAN streams and builds a model of the legitimate data sequences. Then, it detects anomalies by computing the difference between the reconstructed and the respective real sequences. We experimentally evaluated CANnolo on a set of simulated attacks applied over a real-world dataset. We show that our approach outperforms the state-of-the-art model by improving the detection rate and precision.
Project Gallery
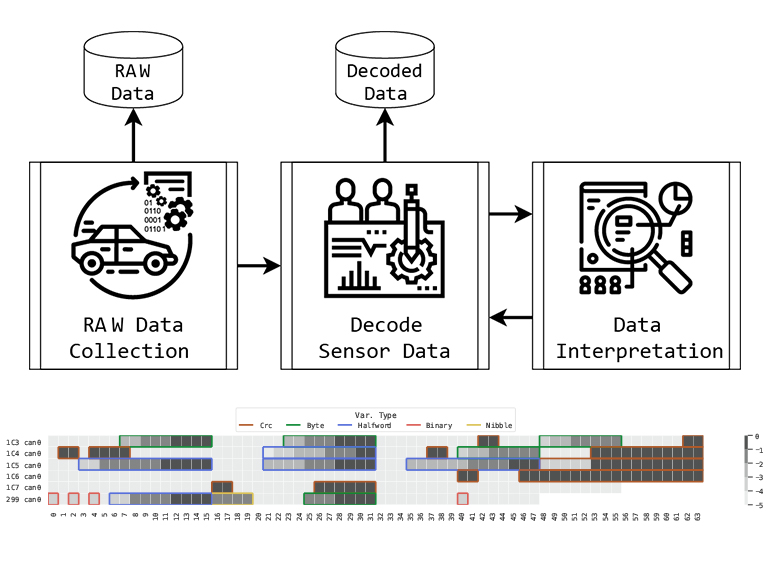
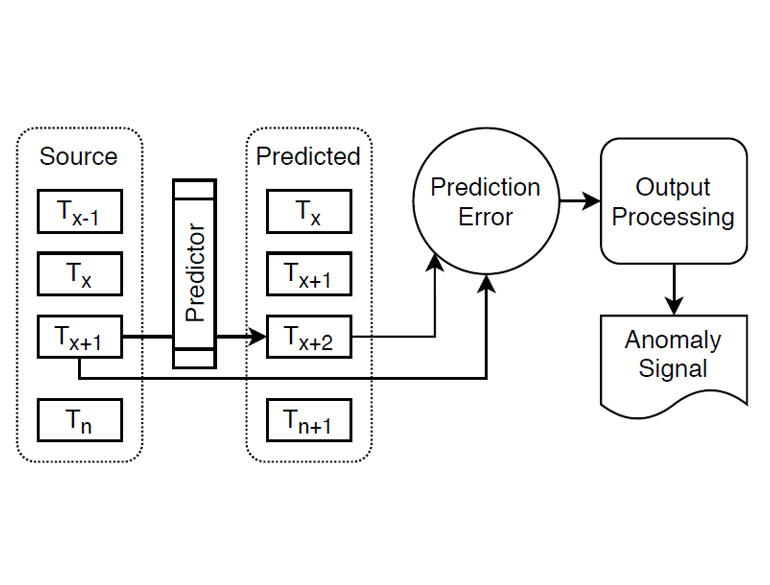