Enhancing DGA-based botnet detection beyond 5G with on-edge machine learning
Executive Summary
Notwithstanding the scientific community's efforts and results, malwares are still wreaking havoc of computer networks; among these threats, botnets are growing at an alarming rate and have been responsible for dangerous attacks. Indeed, in the past five years, notorious botnets such as Mirai, Roboto, or Kraken have been a primary target of the cybersecurity community. However, independently from the purposes of these malwares, the botnets are characterised by a common point of failure, i.e., the communication channel. Infected devices need to reach out to the command and control (C&C) servers to download second-stage infections, perform malicious actions, or await further commands. As the infected devices are already connected to the internet, TCP/IP connections have been widely abused, notwithstanding the providers' efforts in blacklisting IPs and sinkholing fully qualified domain names (FQDNs). Domain generation algorithms (DGAs) have grown to a conventional approach to elude detection algorithms by generating pseudo-random rendezvous-points, i.e., the C&C servers FQDNs. Although many machine learning (ML)-oriented frameworks have been theorised to identify and intercept DGAs, the problem is yet to be solved. As such, this PhD thesis's scope is to analyse the DGAs' outputs, named alghoritmically generated domain names (ADGs), to provide a set of ML tools and privacy-aware methodologies that help identify these evasive patterns.
To be more precise, the objectives achieved throughout this research are twofold. On the one hand, this thesis aims to provide a characterisation of the DGAs aspects, including, among others, a comprehensive survey of previous literary contributions, data sources, and ML-based approaches to detection. On the other hand, it aims to integrate and improve the state-of-the-art by providing methods, strategies, and technologies to enable DGA-based botnet detection at scale. Specifically, signature patterns are identified in malicious ADGs using natural language processing (NLP) techniques and deployed as detection modules on the network's farthest edges.
As a result, this research encompasses literary surveys, theories and frameworks crafting, experiments design and evaluations, and knowledge gaps identification and discussions. Under the compendium modality, the three chapters composing this PhD dissertation are outlined as follows.
- Firstly, a state-of-the-art survey on ML approaches to DGA-based botnet detection; the first chapter reports on supervised and unsupervised algorithms, their features sets, the definition of use cases and experiments, and, ultimately, the outline of multiple research challenges to guide the thesis. Eventually, the experimental findings lay the foundations for ADGs formal and verifiable study.
- Secondly, a comparative analysis of the data sources to power ML frameworks; the second chapter reports on the published datasets by providing a formal comparison and discussion on multiple orthogonal properties. In the same article, the UMUDGA dataset is introduced as the most complete, balanced, and up-to-date collection of DGAs-related data, featuring 50 malware classes for a total of 30+ million FQDNs. Eventually, the exploratory analysis reported in the article suggests that ML solutions to precisely pinpoint the malware variant based on ADGs pattern recognition are feasible.
- Thirdly, a virtualised, proof-of-concept framework where the detection of DGA-based botnets is deployed as a security service on edge; the third chapter compares and examines architectural EAI approaches to enable scalable detection in 5G networks and beyond. In the article, the experimental evaluation demonstrates that ADG detection is not only reasonable and achievable, but it is also plausible to expect to have deployed such detection capabilities on the networks' edges and eventually on the user equipments (UEs)
In summary, the chapters composing this PhD dissertation promote cohesive research exploring, analysing, and ultimately tackling the DGA-based botnets. Following this Ariadne's thread, each chapter is self-contained and provides critical insights on the research challenges from a different perspective; together, these contributions depict a clear description of the research niche summarised in the thesis. However, although conclusive on the explored subjects, some questions mooted by this research remain unsolved. Prime among them is whether it will be feasible to provide anonymous, exchangeable, and trustworthy profiles of ADGs to enable collaborative and federated detection models without harming users' privacy.
Key aspects
Background
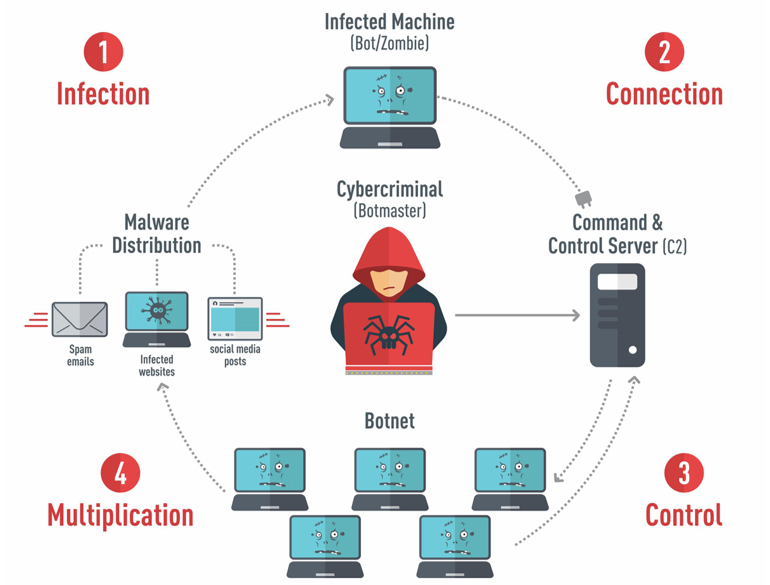
Botnet Lifecycle
A generic botnet presents four steps:
- the infection (where the target device is infected with malware)
- the connection (where the malware tries to reach out for the cybercriminal)
- the control (where the cybercriminal executes commands on the infected device)
- the multiplication (where infected devices spread the malware)
This PhD thesis focuses on the second step to identify newly infected devices during the first attempts to contact the botnet master.
Background
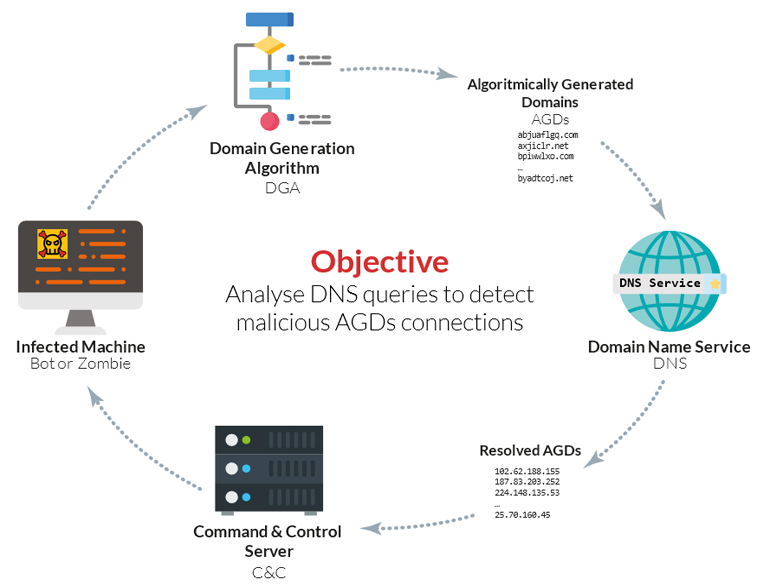
Domain Generation Algorithms
From the cybercriminal perspective, direct IP connections between the infected devices and the C&C servers have been proved ineffective. Hence, they came up with the dynamic generation of FQDNs via pseudo-random generation modules within the malware code. Such modules contain a DGA, a fragment of code that serves to generate pseudo-random domain names that might be registered by the cybercriminals to act as rendezvous-points between C&C servers and infected devices. This intermediate step permits the cybercriminals to generate millions of FQDNs dynamically without the necessity to register all of them, in fact, one available domain name is just enough to permit the connection between the infected device and the C&C servers.
Related works
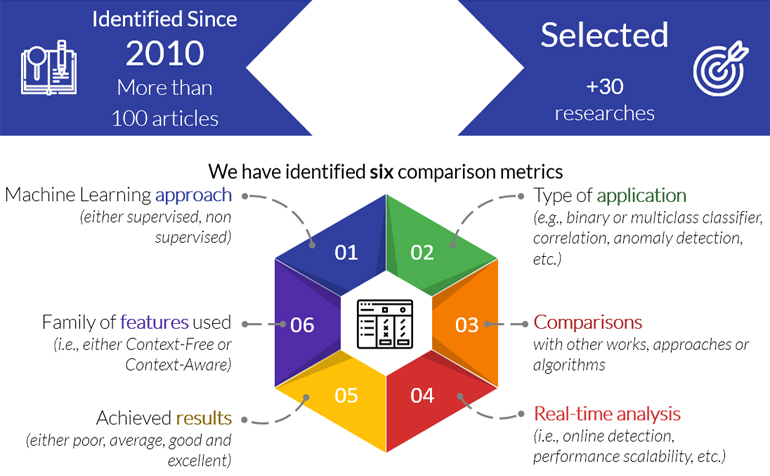
Machine learning approaches
In scientific research, knowing the state-of-the-art is essential. As such, this PhD thesis collected, analysed and presented a survey of the most important published results in the past ten years. Each work has been studied under six prisms:
- the machine learning approach (either supervised, non-supervised, mixed);
- type of application of the machine learning model (anomaly detection, correlation, binary or multiclass classification);
- whether it has been compared with other previous works, and how it relates to them;
- if the framework is designed to perform real-time inference, and if it is built to work at scale;
- claimed achieved results;
- family of features used (either context-free, context-aware, or featureless).
Related works
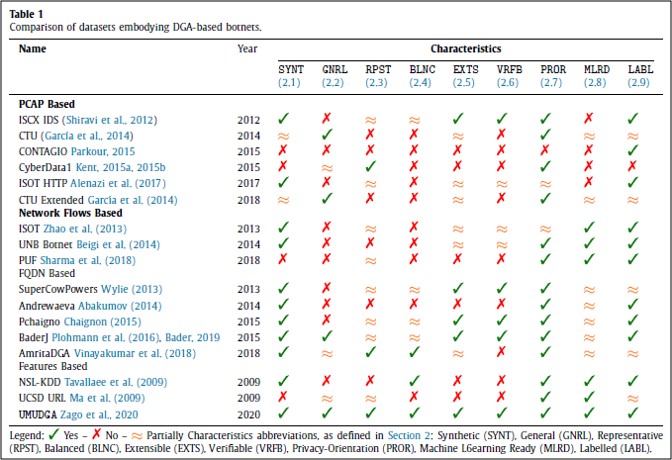
Data sources
To enable the results' reproducibility, it is critical to benchmark the model on publicly available data. One of the most significant contributions of this thesis is to provide an organic comparison of the previously published datasets, and ultimately release a new full-fledged dataset named UMUDGA. Each data source has been evaluated under nine different metrics, explained as follows.
- PR_SYNT — The dataset is artificially created either by generating the samples or by mixing multiple sources.
- PR_GNRL — The dataset covers a wide range of malware families rather than being composed by a few specific examples. To be more precise, the volume of the data is enough to represent a real-world scenario accurately.
- PR_RPST — The dataset includes, for every category, enough instances to accurately reflect the characteristics of the larger population.
- PR_BLNC — The dataset has a comparable number of samples for each category, i.e., the number of instances belonging to a class should not outnumber any other class.
- PR_EXTS — The dataset is publicly available and well documented to enable the research community to extend or combine it with other data sources aiming to improve its reusability.
- PR_VRFB — The data included in the dataset provide enough means to permit the research community to prove the consistency, the accuracy and the genuineness of the data, ideally resulting in a fully reproducible dataset.
- PR_PROR — The dataset has been designed not to include any privacy-harming content or is required for the research community to harm the users' privacy to deploy or include the data in their experiments.
- PR_MLRD — Carefully curated samples compose the dataset. There are no missing values nor unwanted characters. Moreover, the data format is consistent across all the samples, and it is suitable for usage with the leading tools.
- PR_LABL — Each sample is carefully characterised with one or more class attributes, eventually providing a variable granularity of the labels.
Experiments
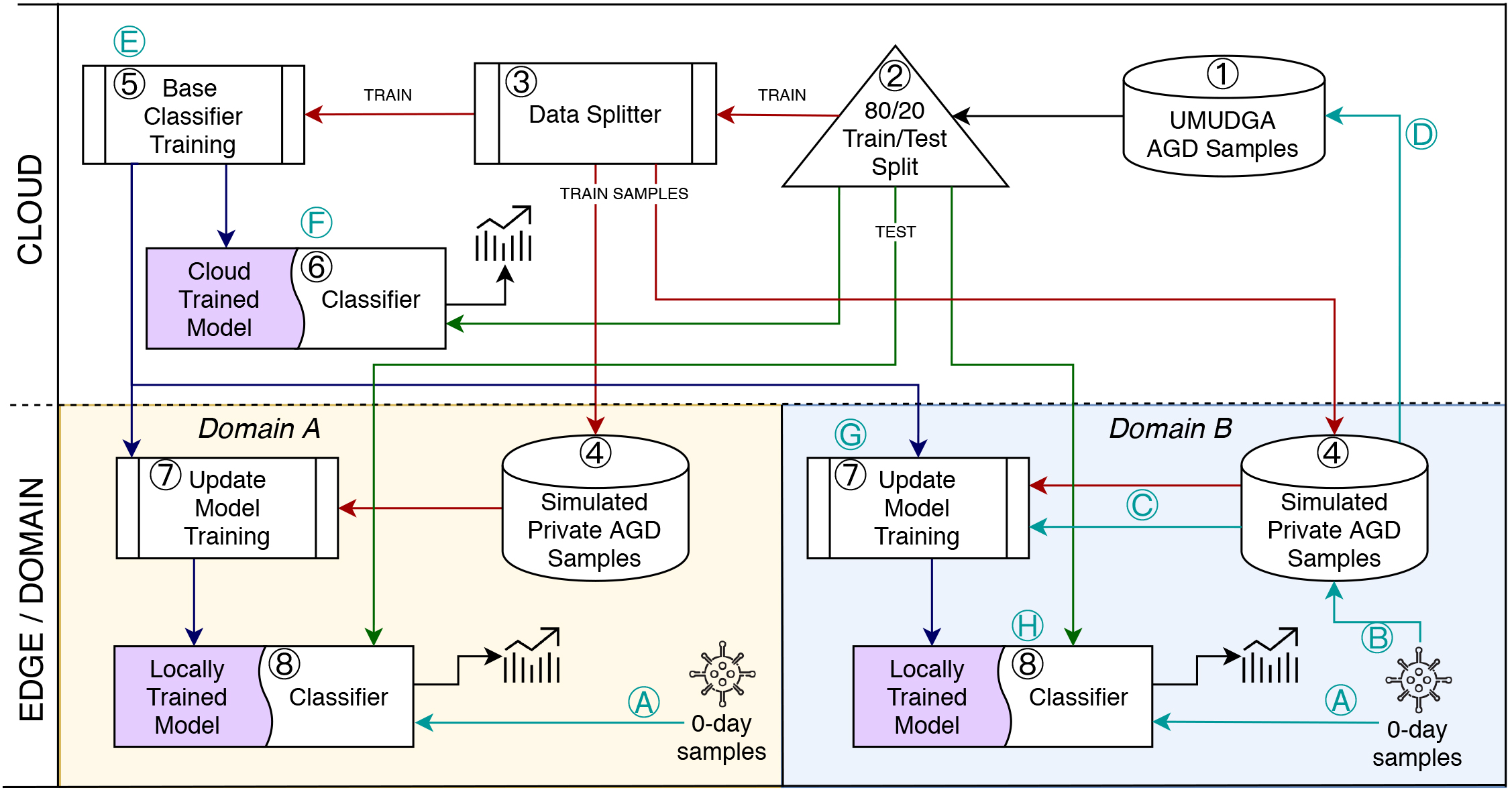
Multiclass identification - SECaaS
Architecture and data flow for the proof-of-concept framework for DGA-based botnet detection.
The figure presents three areas: the Cloud level (on the top half, with a white background) and two edge levels (on the bottom half, with yellow and blue backgrounds). Edges are also identified as "domains" due to the proposed framework's extensibility to an enterprise scenario. In such a case, the company infrastructure might be composed of several edges that rely on a single shared training subcomponent.
The edges, represented as isolated domains, receive a shared and pre-trained detection model that can be augmented with locally available data. Similarly, each domain can willingly provide data or partial models to be included in the shared cloud next training iteration.
Experiments
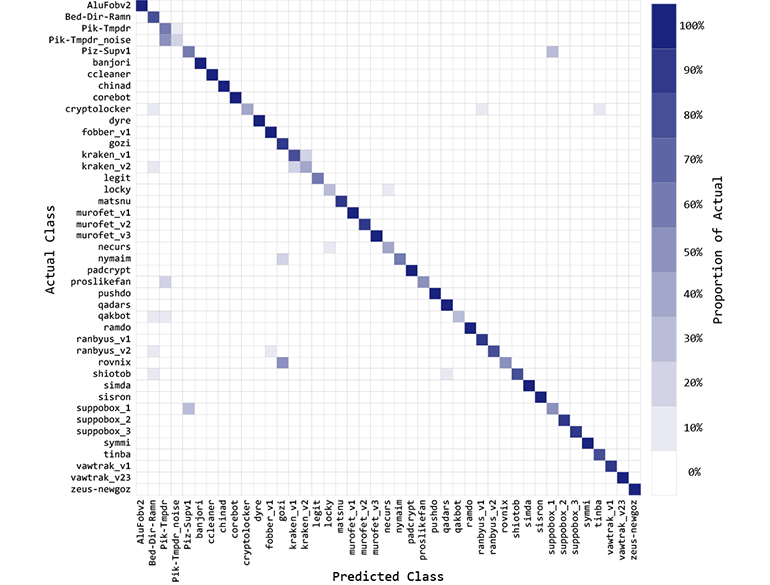
Multiclass identification - Confusion Matrix
The confusion matrix reports the classification results for the UMUDGA malware variants using a Random Forest multiclass classifier. In the figure, each cell represents the classifier's output vs the actual class of the sample; therefore, the darker the colour, the higher the number of records for that combination. It appears clear that although the classifier achieves excellent results in most of the classes, some clusters of classes appears to be difficult to separate.